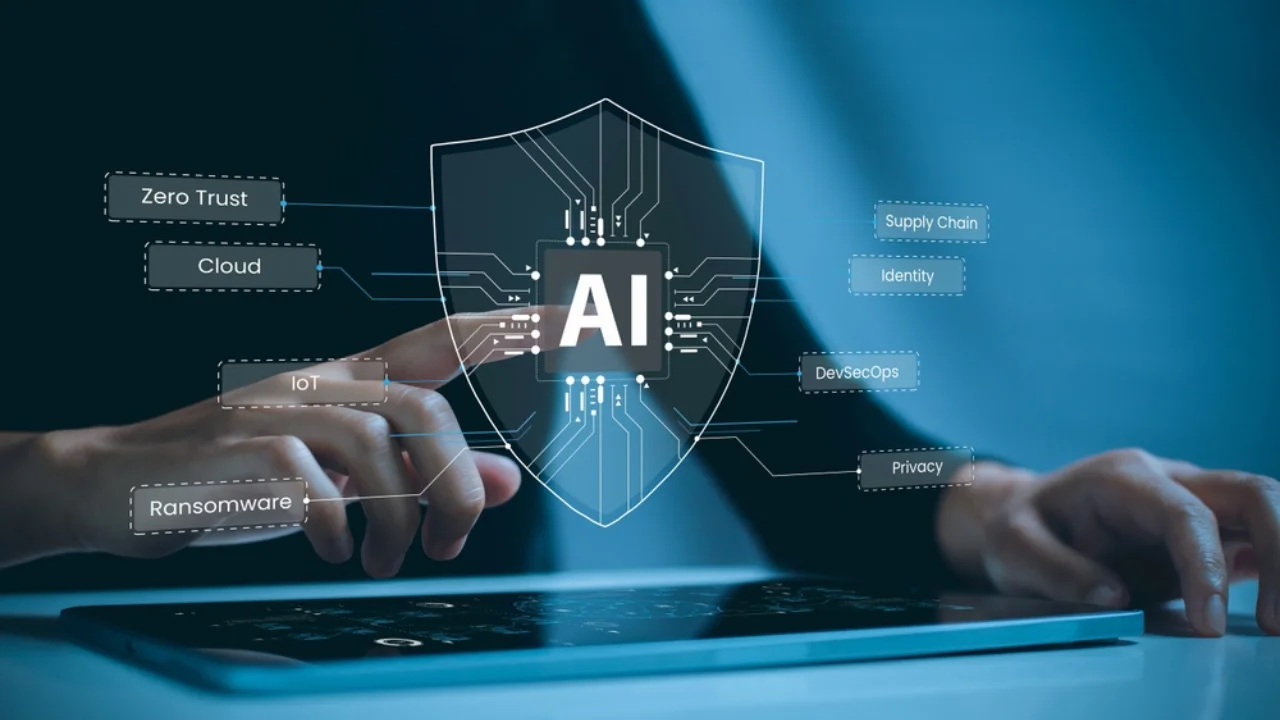
Securing AI Systems – Addressing Vulnerabilities and Threats
It’s imperative to address the vulnerabilities and threats associated in Securing AI systems in order to safeguard sensitive data and prevent potentially catastrophic consequences. AI systems are increasingly being integrated into various aspects of society, from healthcare to finance, raising concerns about security risks. Understanding and implementing robust security measures is crucial to protect against cyberattacks that could compromise the integrity of AI technology. By identifying and mitigating vulnerabilities early on, organizations can ensure the safety and reliability of their AI systems.
Key Takeaways:
- Understand the landscape: Being aware of the potential vulnerabilities and threats in AI systems is crucial to effectively secure them.
- Implement robust security measures: Utilize encryption, access controls, and anomaly detection to protect AI systems from unauthorized access and cyber attacks.
- Regular updates and patches: Stay current with security updates and patches to address any vulnerabilities that may be present in AI systems.
- Conduct security audits: Regularly assess the security posture of AI systems through audits to identify and mitigate any weaknesses.
- Training and awareness: Educate employees on best practices for AI system security to prevent human errors that could lead to security breaches.
AI Security Fundamentals
Defining AI Systems and Their Components
Defining AI systems involves understanding the technology that enables machines to learn from experience, perform human-like tasks, and make decisions. These systems consist of various components such as algorithms, data, models, and interfaces that work together to process information and deliver results.
Basic Principles of AI Security
Their basic principles of AI security revolve around ensuring the confidentiality, integrity, and availability of AI systems and their data. Security measures like encryption, authentication, and access control are imperative to safeguard AI applications against unauthorized access, data breaches, and manipulation.
For instance, lack of proper access controls in AI systems can lead to unauthorized users gaining entry and manipulating data or decisions, posing serious security risks. On the other hand, implementing strong encryption algorithms can help protect sensitive data from being exposed or tampered with.
Types of Vulnerabilities in AI Systems
It is crucial to understand the various types of vulnerabilities that can affect AI systems to effectively address and mitigate potential risks. Some common vulnerabilities include
- Data Poisoning
- Model Tampering
- Adversarial Attacks
- Backdoor Attacks
- Privacy Breaches
. These vulnerabilities can be exploited by malicious actors to manipulate AI systems for their benefit, leading to serious consequences for organizations and individuals. It is crucial to implement robust security measures to protect AI systems from these threats.
Data Poisoning and Model Tampering
For AI systems, data poisoning involves injecting malicious data into training datasets to manipulate the behavior of the models. On the other hand, model tampering occurs when attackers modify the underlying algorithms or parameters of the AI model to generate desired outcomes. These attacks can undermine the integrity and reliability of AI systems, leading to erroneous predictions and decisions.
Adversarial Attacks and Exploits
To safeguard AI systems, it is crucial to address the risks associated with adversarial attacks and exploits. These attacks involve manipulating input data in a way that the AI system produces incorrect outputs, leading to potential security breaches and misinformation. Adversarial attacks can bypass security mechanisms and deceive AI systems, posing significant threats to the integrity of the algorithms and the data they process.
Data poisoning and model tampering can have detrimental effects on the performance and reliability of AI systems, making them vulnerable to manipulation and exploitation. Organizations must prioritize securing their AI systems against these threats to ensure the trustworthiness and effectiveness of their AI-powered solutions.
Strategies for Securing AI Systems
AI System Hardening Techniques
An AI system’s security is crucial in safeguarding against potential threats. One effective strategy is employing system hardening techniques. These techniques involve securing the system by reducing its attack surface, minimizing vulnerabilities, and implementing strict access controls.
The Role of Encryption and Access Control in AI Security
Hardening an AI system involves implementing robust encryption methods and access controls to protect sensitive data and prevent unauthorized access. Encryption ensures that data remains secure during transmission and storage, while access controls restrict system permissions to authorized users only.
Encryption plays a crucial role in securing AI systems as it prevents sensitive data from being compromised in the event of a breach. Access control mechanisms, such as role-based access control (RBAC), further enhance security by ensuring that only users with the necessary credentials can access specific resources and functionalities.
Threat Detection and Response
Monitoring AI Systems for Security Threats
The key to safeguarding AI systems is continuous monitoring for security threats. Monitoring ensures that any anomalies, unauthorized access, or malicious activities are detected and addressed promptly. Implementing robust monitoring mechanisms, such as intrusion detection systems and anomaly detection tools, is crucial to identifying potential security breaches in real-time.
Incident Response and Recovery in AI Ecosystems
Threat detection is only the first step. Having a well-defined incident response plan is crucial for addressing security incidents swiftly and effectively. In AI ecosystems, where the stakes are high, organizations must have a structured approach in place to contain and mitigate the impact of any security breaches.
Response teams should be trained to respond to incidents with speed and efficiency. They need to have clear guidelines on how to isolate affected systems, investigate the root cause of the breach, and implement remediation measures to prevent future incidents. Regular testing and updating of the incident response plan are also crucial to ensure its effectiveness in the face of evolving threats.
Ethical Considerations and Regulatory Compliance
Ethical Implications of AI Security
Your AI system holds a great deal of power in its digital hands. As such, there are ethical implications that must be considered when securing AI systems. Ensuring the confidentiality, integrity, and availability of data is not enough; we must also consider how the decisions made by AI systems can impact individuals and society as a whole. Issues such as bias, privacy invasion, and accountability must be taken into account to build ethically sound AI systems.
Navigating Global AI Security Regulations and Standards
Compliance with global AI security regulations and standards is important for organizations working with AI technologies. Regulatory bodies worldwide are increasingly focusing on AI security due to the potential risks associated with AI deployment. Navigating this complex landscape requires a deep understanding of the various regulations and standards that apply to AI systems, spanning data protection laws, industry-specific regulations, and international standards.
Ethical considerations must guide organizations in not only meeting regulatory requirements but also exceeding them to ensure the ethical use and deployment of AI systems. Proactively addressing ethical considerations in AI security can help organizations build trust with their customers, regulators, and the general public, positioning them as responsible stewards of AI technology.
Securing the Future of AI
Advancements in Securing AI Systems
On the quest to fortify AI systems, advancements in AI security technologies have been pivotal. Innovations such as robust encryption techniques, secure multi-party computation, and AI-driven cybersecurity tools have propelled the defense mechanisms against potential threats. These technologies are designed to proactively identify vulnerabilities and thwart malicious activities that could compromise the integrity of AI systems.
Preparing for Emerging Threats in an Evolving Landscape
Emerging technologies bring forth new possibilities, but also new risks. As the AI landscape evolves, so do the threats that seek to exploit vulnerabilities within AI systems. Understanding the potential risks and preparing for emerging threats is vital to safeguarding AI technologies. Threats such as adversarial attacks, data poisoning, and model inversion attacks pose serious risks to the security of AI systems and must be addressed through robust security measures.
Summing up
Conclusively, securing AI systems is paramount in addressing vulnerabilities and threats that could potentially compromise critical systems and data. By implementing robust security measures, such as encryption, authentication, and monitoring, organizations can better protect themselves against cyber threats. Regularly updating software and conducting thorough risk assessments can also help in identifying and addressing potential vulnerabilities. As AI technology continues to advance, it is crucial for organizations to stay vigilant and take proactive steps to safeguard their systems from potential security breaches.