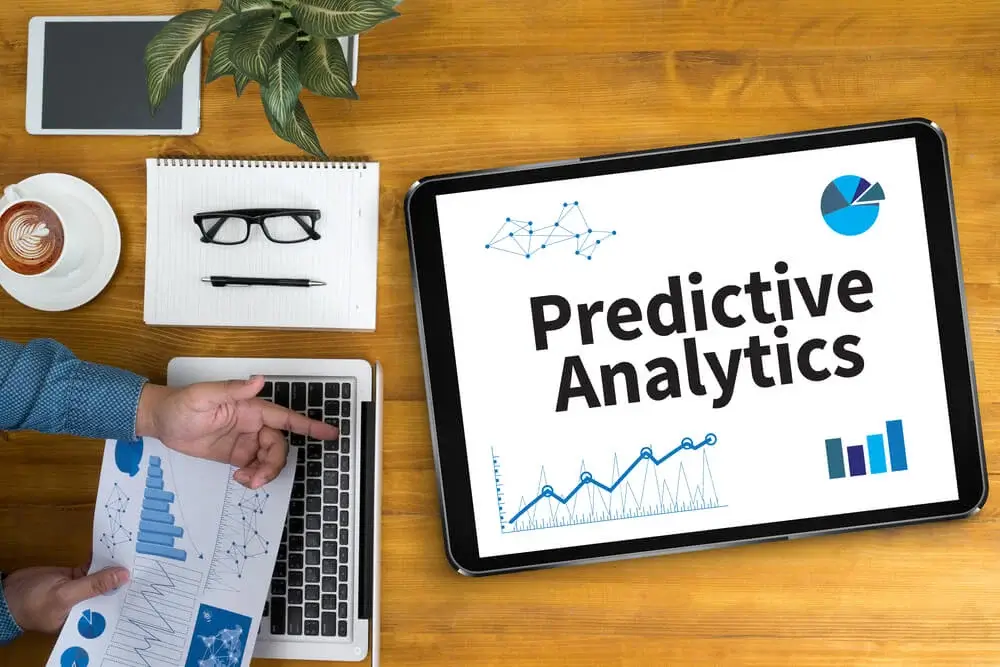
Unlocking Insights with Predictive Analytics: Applications in Business and Beyond
Data-driven decision-making and predictive analytics stands as a beacon of foresight. Defining predictive analytics entails employing statistical algorithms and machine learning techniques to forecast future outcomes based on historical data patterns. Unlike descriptive analytics that focus on understanding past events or diagnostic analytics that pinpoints reasons behind occurrences, predictive analytics anticipates what might happen next. Its importance lies in its ability to empower organizations with actionable insights, enhancing decision-making processes and providing a competitive advantage in dynamic markets. Whether in business strategies or broader societal applications, predictive analytics illuminates pathways to success.
The Process of Predictive Analytics
Data Collection and Preparation
In the realm of predictive analytics, the journey begins with data collection and preparation. Identifying relevant data sources is crucial, as the quality and scope of data directly impact the accuracy of predictions. This stage involves gathering structured and unstructured data from various internal and external sources, including customer databases, social media platforms, and IoT devices. Once collected, the data undergoes rigorous cleaning and preprocessing to remove inconsistencies, outliers, and missing values. Techniques such as data imputation, normalization, and feature engineering are employed to ensure data integrity and suitability for analysis.
Model Building
With clean and well-prepared data in hand, the next step in the predictive analytics process is model building. This involves selecting appropriate algorithms tailored to the specific prediction task at hand. Whether it’s regression models for numerical predictions or classification algorithms for categorical outcomes, the choice of algorithm depends on factors such as the nature of the data, the complexity of the problem, and the desired level of interpretability. Once selected, the model undergoes rigorous training, where it learns patterns and relationships from the historical data through iterative optimization processes.
Evaluation and Validation
After the model has been trained, it is essential to evaluate its performance and validate its predictive capabilities. Assessing model performance involves comparing the predictions generated by the model against actual outcomes using metrics such as accuracy, precision, recall, and F1-score. This step helps gauge the model’s effectiveness in capturing underlying patterns and making accurate predictions. Furthermore, fine-tuning for accuracy is performed by adjusting model parameters, feature selection, or hyperparameter optimization to optimize predictive performance.
Applications in Business
In the realm of business, predictive analytics serves as a powerful tool for driving strategic decision-making and gaining a competitive edge.
Customer Relationship Management
In the dynamic landscape of Customer Relationship Management (CRM), predictive analytics plays a pivotal role in predicting customer churn. By analyzing historical customer data and identifying patterns indicative of potential churn, businesses can proactively implement retention strategies to minimize customer attrition. Moreover, predictive analytics enables personalizing marketing campaigns by segmenting customers based on their preferences, behaviors, and purchasing patterns. This targeted approach enhances customer engagement and fosters long-term loyalty.
Financial Forecasting
In the realm of financial forecasting, predictive analytics offers invaluable insights into predicting market trends and identifying lucrative investment opportunities. By analyzing market indicators, economic data, and historical performance, businesses can anticipate shifts in consumer demand, industry trends, and market dynamics. Armed with this foresight, organizations can make informed investment decisions, capitalize on emerging opportunities, and mitigate potential risks.
Supply Chain Management
It also revolutionizes Supply Chain Management (SCM) by enabling businesses to accurately forecast demand and optimize inventory levels. By analyzing historical sales data, market trends, and external factors such as seasonality and economic fluctuations, organizations can anticipate future demand patterns and adjust inventory levels accordingly. This proactive approach minimizes stockouts, reduces excess inventory costs, and enhances overall supply chain efficiency.
Applications Beyond Business
While predictive analytics is often associated with business applications, its impact extends far beyond corporate boardrooms, reaching into critical sectors such as healthcare, transportation, and environmental science.
Healthcare
In healthcare, analytics plays a transformative role in predicting patient outcomes. By analyzing vast amounts of patient data, including medical history, lab results, and lifestyle factors, predictive models can forecast the likelihood of future health events such as hospital readmissions or disease progression. Furthermore, predictive analytics facilitates personalized medicine by tailoring treatment plans and interventions based on individual patient characteristics and predicted outcomes. This targeted approach improves patient outcomes, enhances quality of care, and reduces healthcare costs.
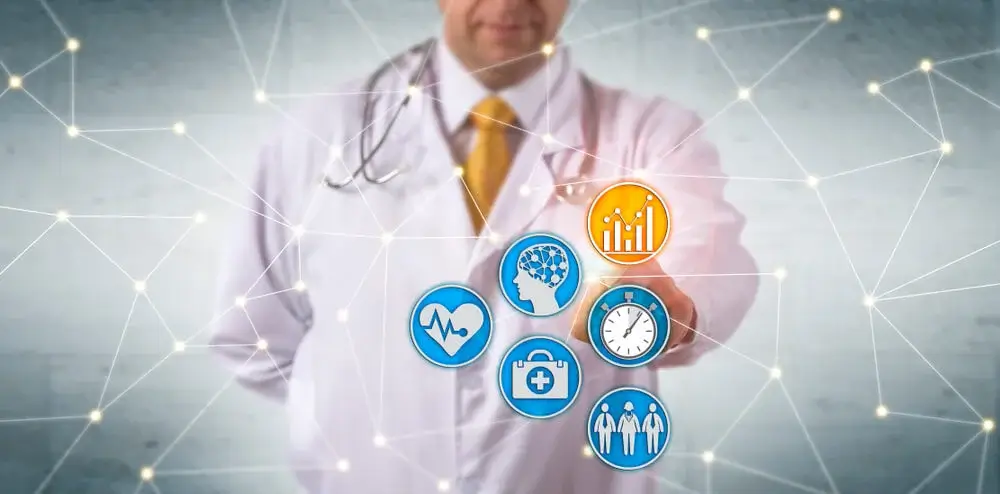
Transportation
In transportation, this analytics revolutionizes predicting traffic patterns and optimizing routes for efficiency. By analyzing historical traffic data, weather patterns, and real-time sensor data from vehicles and infrastructure, predictive models can forecast traffic congestion and identify optimal routes for commuters and freight carriers. Additionally, predictive analytics enables transportation agencies to proactively manage traffic flow, alleviate congestion hotspots, and improve overall transportation efficiency, leading to reduced travel times and fuel consumption.
Environmental Science
Predictive analytics also plays a critical role in environmental science by predicting natural disasters and monitoring climate change. By analyzing climate data, satellite imagery, and geological information, predictive models can forecast events such as hurricanes, earthquakes, and wildfires, enabling authorities to issue timely warnings and implement preventive measures to mitigate risks and protect communities. Furthermore, predictive analytics aids in monitoring climate change by analyzing long-term environmental data and identifying trends and patterns indicative of climate variability and global warming. This information informs policymakers, scientists, and stakeholders, guiding efforts to develop sustainable environmental policies and mitigate the impact of climate change on ecosystems and human populations.
Challenges and Limitations
Despite its transformative potential, the widespread adoption of predictive analytics is not without its challenges and limitations.
Data Quality and Availability
One of the foremost challenges in predictive analytics is data quality and availability. Dealing with incomplete or biased data can significantly impact the accuracy and reliability of predictive models. Incomplete datasets may lead to erroneous conclusions, while biased data can perpetuate disparities and inequities. Moreover, ensuring data privacy and security is paramount, particularly when dealing with sensitive information such as personal health records or financial data. Striking a balance between data accessibility and privacy protection is essential to foster trust and compliance in predictive analytics initiatives.
Model Interpretability
Another challenge lies in model interpretability. While predictive models can generate accurate predictions, understanding the rationale behind these predictions is crucial for gaining stakeholders’ trust and confidence. Addressing potential biases in algorithms and ensuring transparency in model decision-making processes are essential steps in enhancing interpretability and accountability. By providing insights into how predictive models arrive at their conclusions, organizations can improve decision-making and mitigate the risk of unintended consequences.
Implementation Complexity
Integrating predictive analytics into existing systems poses a significant challenge due to implementation complexity. Organizations must navigate technical hurdles and organizational barriers to successfully deploy predictive models in real-world settings. This may involve integrating predictive analytics into existing systems such as customer relationship management platforms or enterprise resource planning software. Additionally, overcoming resistance to change from stakeholders accustomed to traditional decision-making approaches requires effective communication, training, and change management strategies.
Future Trends and Innovations
As predictive analytics continues to evolve, several future trends and innovations are poised to shape its trajectory.
AI and Machine Learning Advancements
Advancements in AI and machine learning are propelling predictive analytics to new heights. Leveraging deep learning techniques, such as neural networks, enables more accurate predictions by uncovering intricate patterns in complex datasets. Moreover, there is a growing interest in exploring the potential of unsupervised learning, which allows algorithms to identify hidden structures and relationships in data without the need for labeled examples. These advancements promise to enhance the predictive capabilities of analytics models across various domains.
Ethical Considerations
As predictive analytics becomes more pervasive, addressing ethical considerations is paramount. Organizations must prioritize developing guidelines for the responsible use of predictive analytics to ensure transparency, fairness, and accountability in decision-making processes. Additionally, mitigating concerns about algorithmic bias is essential to prevent discriminatory outcomes and uphold ethical standards. By implementing robust ethical frameworks, organizations can build trust and credibility in their predictive analytics initiatives.
Democratization of Predictive Analytics
The democratization of this analytics is another emerging trend, aiming to make tools and resources more accessible to non-experts. By simplifying complex algorithms and workflows, businesses and individuals can harness the power of predictive analytics without specialized technical knowledge. This democratization empowers organizations to leverage data-driven insights to drive innovation, improve efficiency, and gain a competitive edge in the marketplace.
Conclusion
In conclusion, predictive analytics stands as a beacon of insight, guiding organizations towards informed decision-making and strategic planning. Its significance lies in its ability to unravel hidden patterns and forecast future trends, unlocking opportunities for growth and innovation. I encourage exploration of its applications in various fields, from healthcare to environmental science, where it can drive positive change and address complex challenges. However, it’s crucial to emphasize the need for responsible and ethical use of predictive analytics, ensuring fairness, transparency, and respect for privacy rights. With careful consideration and conscientious implementation, predictive analytics can truly revolutionize business and beyond.