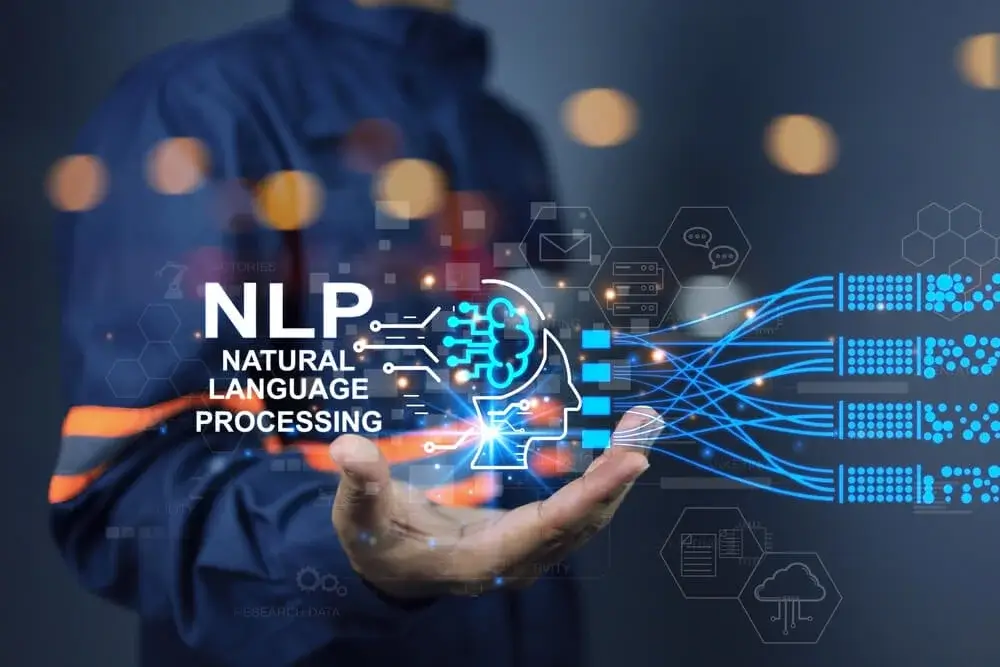
Natural Language Processing in the Real World: Applications and Challenges
Today the prominence of Natural Language Processing in the Real World: Applications and Challenges has skyrocketed. From chatbots to language translation, natural language processing (NLP) has become an indispensable tool in our daily lives. NLP involves the intersection of linguistics, computer science, and artificial intelligence, empowering machines to understand, interpret, and generate human language. As we delve deeper into its applications and challenges, let’s explore the transformative potential of NLP.
Real-world Applications of NLP
In today’s digital landscape, Natural Language Processing in the Real World: Applications and Challenges is reshaping various industries and revolutionizing the way we interact with technology. Let’s explore some of the key applications of NLP and how they are transforming different aspects of our lives.
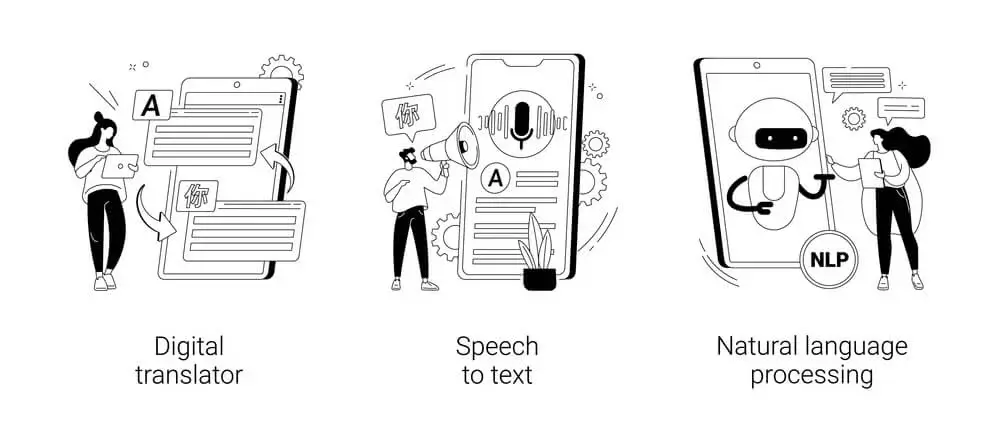
Sentiment Analysis
Analyzing sentiments on social media allows businesses to gain insights into public opinion, brand perception, and trends.
Customer feedback analysis for businesses enables companies to understand customer satisfaction levels, identify areas for improvement, and make data-driven decisions to enhance their products or services.
Language Translation
Google Translate and other translation services have made multilingual communication seamless, facilitating cross-border interactions in business, diplomacy, and personal communication.
Multilingual communication in business and diplomacy is essential for fostering collaboration, breaking down language barriers, and expanding global reach.
Text Summarization
Automatic summarization of articles and documents saves time and resources by condensing large volumes of text into concise summaries.
Enhancing efficiency in information retrieval allows users to quickly access relevant information without having to sift through lengthy documents or articles.
Chatbots and Virtual Assistants
Customer service automation streamlines support processes, reduces response times, and improves customer satisfaction by providing instant assistance.
Personal assistants like Siri, Alexa, and Google Assistant enhance productivity, facilitate voice-based interactions, and perform tasks such as setting reminders, answering questions, and controlling smart devices.
Named Entity Recognition
Extracting entities such as names, dates, and locations from text enables organizations to organize and analyze large datasets more efficiently.
Applications in information retrieval and data analysis help researchers, analysts, and businesses uncover valuable insights and patterns hidden within text data.
Challenges in NLP Implementation
Implementing Natural Language Processing in the Real World: Applications and Challenges comes with its fair share of obstacles that organizations must overcome to harness the full potential of NLP technology.
Ambiguity and Polysemy
Handling words with multiple meanings poses a challenge as NLP systems must discern the correct interpretation based on context.
Achieving contextual understanding in language processing is crucial for accurately interpreting ambiguous phrases or sentences.
Data Quality and Quantity
The availability of labeled training data is essential for training NLP models effectively, but obtaining high-quality labeled data can be time-consuming and costly.
Bias and representativeness in training datasets can lead to skewed results and limit the generalizability of NLP models.
Domain-specific Challenges
Tailoring NLP models for specific industries or domains requires customization to account for domain-specific vocabulary, jargon, and language conventions.
Vocabulary and terminology variations across domains present challenges in ensuring NLP models can accurately process and understand specialized language.
Language Complexity
Dealing with slang, colloquialisms, and informal language poses challenges as NLP systems must be able to understand and interpret non-standard language usage.
Understanding dialects and regional variations adds complexity to language processing tasks, requiring NLP models to be trained on diverse linguistic datasets.
Ethical Considerations
Privacy concerns in text analysis arise from the potential for NLP systems to inadvertently expose sensitive information contained within textual data.
Bias and fairness in NLP algorithms are ethical considerations that must be addressed to ensure NLP systems do not perpetuate or amplify existing biases in society.
Future Directions in Natural Language Processing
As Natural Language Processing in the Real World: Applications and Challenges continues to evolve, several exciting avenues are emerging that promise to reshape the landscape of NLP technology in the future.
Advancements in Deep Learning
Transformer architectures and attention mechanisms have revolutionized NLP by enabling models like BERT and GPT to achieve state-of-the-art performance in language understanding tasks.
These advancements have led to enhanced performance in tasks such as sentiment analysis, language translation, and text summarization, pushing the boundaries of what NLP systems can accomplish.
Multimodal NLP
Integrating text with other modalities like images and audio is a promising area of research that seeks to enrich language understanding by incorporating additional sensory information.
Advancements in understanding and generating multimodal content hold the potential to revolutionize applications such as image captioning, video understanding, and virtual assistants.
Explainable AI in Natural Language Processing
Interpretable models are essential for transparency and trust in NLP systems, allowing users to understand how decisions are made and providing insights into model behavior.
Addressing the black box nature of deep learning models is crucial for making NLP technology more accessible and trustworthy, especially in sensitive applications such as healthcare and finance.
Contextualized Representations
Context-aware language understanding and generation aim to capture the nuances of language by considering the surrounding context and incorporating contextual information into NLP models.
Improving naturalness and coherence in text generation is essential for creating more human-like responses in chatbots, virtual assistants, and other conversational AI systems.
Ethical and Responsible Natural Language Processing
Fairness, transparency, and accountability are paramount in ensuring that NLP systems are deployed responsibly and ethically, particularly in applications that impact individuals and society.
Mitigating biases and ensuring inclusivity in language processing is essential for building fair and equitable NLP systems that serve diverse user populations.
Conclusion
In conclusion, Natural Language Processing in the Real World: Applications and Challenges has transformed the way we interact with technology and communicate in today’s digital age. We’ve explored key applications of NLP, from sentiment analysis to language translation, and discussed the challenges and future directions shaping the field. As NLP continues to advance, it will play an increasingly vital role in shaping the future of technology and communication. Its impact on various industries and its potential to address complex societal challenges underscore the importance of NLP in driving innovation and shaping our digital world.