Many advancements in the field of artificial intelligence have been made possible by the evolution of hardware used in AI systems. As technology continues to progress, so does the complexity and capabilities of the hardware that powers these intelligent systems. Understanding the journey from lines of code to physical creations is crucial in grasping the potential and limitations of AI technology. This blog post dives into the transformative impact of hardware innovations on AI systems, shedding light on the key milestones that have shaped the current landscape of artificial intelligence hardware.
Key Takeaways:
- Hardware evolution: The hardware used in AI systems has evolved significantly over the years, from generic processors to specialized hardware like GPUs and TPUs, to meet the computational demands of AI algorithms.
- Efficiency and speed: Specialized hardware like GPUs and TPUs offer increased efficiency and speed in performing AI tasks compared to traditional processors, enabling faster training and inference of neural networks.
- Co-design approach: The trend towards a co-design approach, where hardware and software are developed in tandem to optimize performance, is gaining traction in the field of AI hardware development.
- Customized solutions: Companies are increasingly investing in designing custom hardware solutions tailored to their specific AI workloads, aiming to achieve higher performance and energy efficiency.
- Future trends: The future of AI hardware is likely to involve a mix of specialized and general-purpose processors, as well as more emphasis on energy-efficient designs to support the growing demands of AI applications.
Historical Context and the Role of Hardware
The Birth of Artificial Intelligence
On the brink of the digital era, the concept of Artificial Intelligence (AI) emerged as a possibility. In the mid-20th century, pioneers like Alan Turing and John McCarthy laid the foundation for AI by proposing the idea of machines with the ability to think and learn like humans.
Milestones in AI Hardware Development
Historical advancements in AI hardware development have been pivotal in shaping the capabilities of AI systems. From the first computers capable of performing logical operations to the advent of specialized hardware like GPUs and TPUs designed for accelerating AI tasks, the evolution of hardware has played a crucial role in the growth of AI.
To research deeper into the history of AI hardware development, it’s important to highlight the transition from general-purpose computing to specialized hardware architectures specifically optimized for AI workloads. The development of Graphics Processing Units (GPUs) and Tensor Processing Units (TPUs) has significantly enhanced the performance and efficiency of AI systems, enabling faster processing of complex algorithms and models.
Technological Advancements in AI Hardware
From CPUs to GPUs and TPUs
Now, AI hardware has evolved from using traditional Central Processing Units (CPUs) to more specialized Graphics Processing Units (GPUs) and Tensor Processing Units (TPUs).
Neural Network Processors and Custom ASICs
With the proliferation of deep learning and complex neural networks, the demand for more efficient hardware led to the development of Neural Network Processors and Custom Application-Specific Integrated Circuits (ASICs).
This evolution has sparked a race to create more powerful and efficient hardware tailored specifically for AI tasks. Custom ASICs provide a streamlined approach to executing neural network operations, significantly improving performance and energy efficiency compared to general-purpose processors.
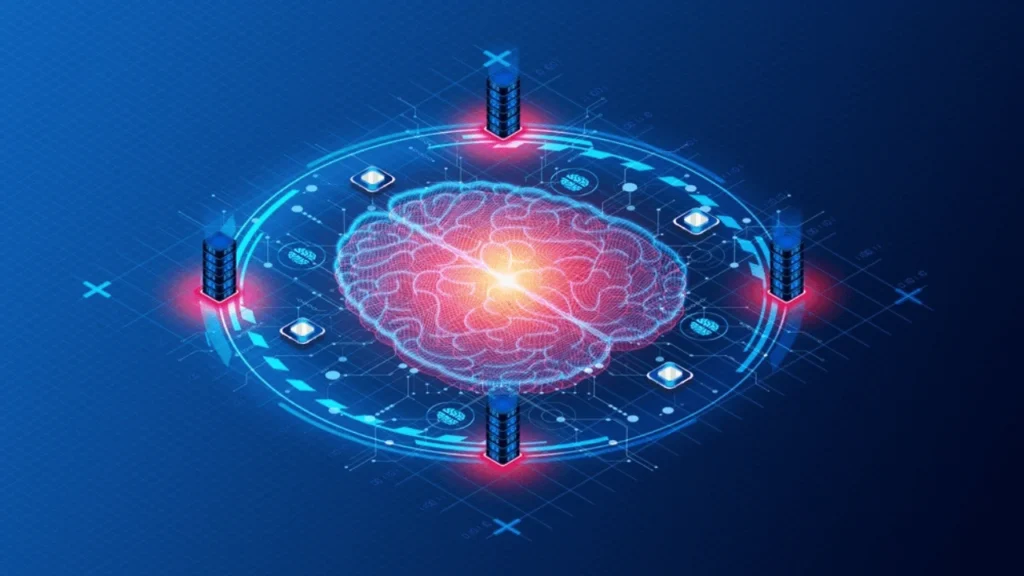
Challenges and Innovation in AI Hardware Design
Power Consumption and Efficiency
Challenges in AI hardware design often revolve around power consumption and efficiency. As AI systems become more complex and demanding, there is a growing need for hardware that can handle intensive computational tasks while remaining energy-efficient. The challenge lies in finding the balance between performance and power consumption to create more sustainable and cost-effective AI hardware solutions.
Scalability and Integration
An important aspect of AI hardware design is scalability and integration. The ability to scale AI systems according to workload demands and seamlessly integrate different components is vital for optimal performance. For instance, the integration of CPUs, GPUs, and specialized accelerators can significantly boost the processing power of AI systems and enable faster data processing for complex algorithms.
The Future of AI in Hardware Systems
Emerging Technologies and Trends
All breakthroughs and advancements in AI hardware are paving the way for precisely tailored systems that can meet the evolving demands of AI applications. Technologies such as neuromorphic computing, quantum processors, and photonic computing are at the forefront of innovation in the AI hardware domain. These technologies offer the promise of higher efficiency, faster processing speeds, and increased computational power that could potentially revolutionize the AI landscape.
The Impact on AI Capabilities and Applications
An additional impact of these advancements in AI hardware is the potential to enhance AI capabilities across various industries. As hardware becomes more efficient and powerful, AI systems can handle more complex tasks, process larger amounts of data, and deliver more accurate and timely results. This could lead to significant improvements in healthcare, finance, autonomous vehicles, and many other sectors.
Another crucial aspect of the impact of advancements in AI hardware is the potential for accelerating the development and deployment of AI solutions. Faster and more efficient hardware can reduce the time and resources required for training AI models, enabling quicker innovation and deployment of AI applications. This could lead to a faster pace of technological advancement and a more widespread integration of AI into various aspects of our everyday lives.
To wrap up
Taking this into account, we have observed the remarkable evolution of hardware in AI systems, from early analog computers to the current era of sophisticated neural networks. The advancements in hardware technology have played a significant role in pushing the boundaries of what AI systems can achieve, enabling capabilities that were once only seen in science fiction. As we continue to progress into the future, it is clear that the development of hardware will remain a crucial component in further enhancing the power and efficiency of AI systems, paving the way for groundbreaking innovations in various industries and sectors.